TensorFlow 的卷积操作 Python 实现
这两天看到了一个卷积的实现代码,挺有意思,自己照着他的代码复写了一遍,算是加深下对卷积的理解( 略微改动了下代码以便更加清晰的进行两种卷积的对比)。
先上原理图:
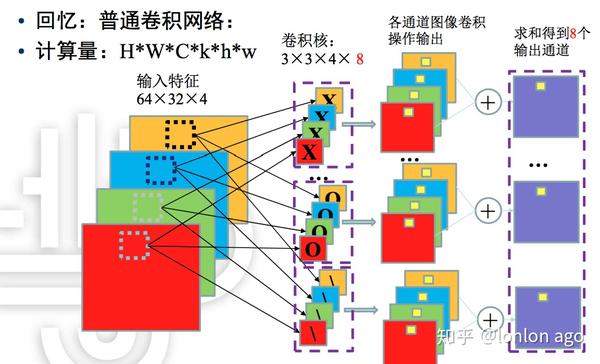
再上代码,解释都写在代码里面了,里面有两部分代码,分别用 TensorFlow 实现卷积和自己代码实现卷积。
#coding:utf-8
import numpy as np
import tensorflow as tf
#输入,shape=[c,h,w] 2*5*5 ,
# 因为我们使用的列表或者数组都只能看到最内层的shape,比如这里只能明显看到5*5的矩阵,我们如果要在
# tensorflow 里面进行操作,必须是四维的数据,比如 batch_size*h*w*chanel 这样的,那么我们能明显看到的数据就是
# 5*chanel 的部分,所以为了符合 tensorflow 的要求,我们需要对数据进行形状转换,先把它转换为 5*5*2,
# 再转换为 1*5*5*2
input_data=np.array([
[[1,0,1,2,1],
[0,2,1,0,1],
[1,1,0,2,0],
[2,2,1,1,0],
[2,0,1,2,0]],
[[2,0,2,1,1],
[0,1,0,0,2],
[1,0,0,2,1],
[1,1,2,1,0],
[1,0,1,1,1]],
]).astype(np.float32)
#卷积核,shape=[in_c,k,k]=[2,3,3] , 将它转换为 3*3*2*1
weights_data=np.array([
[[ 1, 0, 1],
[-1, 1, 0],
[ 0,-1, 0]],
[[-1, 0, 1],
[ 0, 0, 1],
[ 1, 1, 1]]
]).astype(np.float32)
def chw2hwc(chw_tensor):
[c, h, w] = chw_tensor.shape
cols = []
for i in range(c):
line = np.reshape(chw_tensor[i], [h*w, 1])
cols.append(line)
inputd = np.concatenate(cols, 1) # 25*2 如果是0轴的话,就是50*1
inputd = np.reshape(inputd, [h, w, c])
return inputd
def hwc2chw(tensor):
[h, w, c] = tensor.shape
cs = []
for i in range(c):
channel = np.expand_dims(tensor[:,:,i], 0) # 1*h*w
# print(channel.shape)
cs.append(channel)
inputd = np.concatenate(cs)
return inputd
# print(chw2hwc(input_data))
def tensorflow_conv_example():
input_data_fixed = chw2hwc(input_data) # 2 5 5 > 5 5 2
input_tensor = tf.Variable(input_data_fixed, tf.float32, name='input')
input_tensor = tf.expand_dims(input_tensor, 0) # 5 5 2 > 1 5 5 2
print(input_tensor.get_shape())
weights_data_fixed = chw2hwc(weights_data) # 2 3 3 > 3 3 2
input_weight = tf.Variable(weights_data_fixed, tf.float32, name='weight')
input_weight = tf.expand_dims(input_weight, 3) # 3 3 2 > 3 3 2 1
print(input_weight.get_shape())
conv = tf.nn.conv2d(input_tensor, input_weight, strides=[1,1,1,1], padding='SAME')
init = tf.global_variables_initializer()
sess = tf.Session()
sess.run(init)
conv_result = sess.run(conv)
print('input_tensor:',sess.run(input_tensor))
# print(conv_result)
print('tensorflow result:\n',hwc2chw(conv_result[0]))
tensorflow_conv_example()
计算单个通道的卷积操作的函数,这里其实默认设置了padding为全补0, 步长为1的卷积
:param fm: 输入数据的一层 [ h, w ]
:param kernel: 卷积核的一层 [ k, k ]
:return:
[h, w] = fm.shape
[k, _] = kernel.shape
r = int(k/2) # 卷积核的半径,也就是卷积核覆盖的范围和视野
print('fm.shape:', fm.shape, 'kernel.shape:',kernel.shape, 'r:',r)
# 对数据周边做全0填充,这里对每个边缘都补充了1行或1列数量的0,加起来就是行和列都补充2
# 这个数目主要是由stride 的数目决定的
padding_fm = np.zeros([h+2, w+2], np.float32)
rs = np.zeros([h, w], np.float32)
padding_fm[1:h+1, 1:w+1] = fm
# 每一个步长做卷积计算
for i in range(1, h+1):
for j in range(1, w+1):
roi = padding_fm[i-r:i+r+1, j-r:j+r+1] #
rs[i-1][j-1] = np.sum(roi * kernel)
return rs
def my_conv_example(input_tensor, weight):
自己计算卷积的示例,因为使用 batch_size 为 1,所以数据维度就只有3维了,
并且不需要使用 chw > whc 的转换了
:param input_tensor: 输入数据 [c,h,w]
:param weight: 卷积核 [c,k,k]
:return:
[c, h, w] = input_tensor.shape
[_, k, _] = weight.shape
output = np.zeros([h, w], np.float32)
# 对每个通道的数据进行卷积操作,并将得到的结果累加求和
for i in range(c):
f_map = input_tensor[i]
w = weight[i] # 每一层 feature map 对应一个卷积核
rs = conv_op(f_map, w)